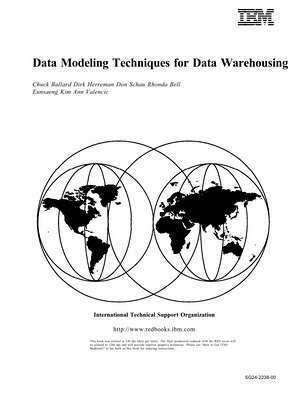
Contents
Figures . . . . . . . . . . . . . . . . . . . . . . . . . . . . . . . . . . . . . . . . . . . ix
Tables . . . . . . . . . . . . . . . . . . . . . . . . . . . . . . . . . . . . . . . . . . . xi
Preface . . . . . . . . . . . . . . . . . . . . . . . . . . . . . . . . . . . . . . . . . xiii
The Team That Wrote This Redbook . . . . . . . . . . . . . . . . . . . . . . . . xiii
Comments Welcome . . . . . . . . . . . . . . . . . . . . . . . . . . . . . . . . . xiv
Chapter 1. Introduction . . . . . . . . . . . . . . . . . . . . . . . . . . . . . . . . . 1
1.1 Who Should Read This Book . . . . . . . . . . . . . . . . . . . . . . . . . . . 2
1.2 Structure of This Book . . . . . . . . . . . . . . . . . . . . . . . . . . . . . . . 2
Chapter 2. Data Warehousing . . . . . . . . . . . . . . . . . . . . . . . . . . . . . 5
2.1 A Solution, Not a Product . . . . . . . . . . . . . . . . . . . . . . . . . . . . . 5
2.2 Why Data Warehousing? . . . . . . . . . . . . . . . . . . . . . . . . . . . . . 5
2.3 Short History . . . . . . . . . . . . . . . . . . . . . . . . . . . . . . . . . . . . 6
Chapter 3. Data Analysis Techniques . . . . . . . . . . . . . . . . . . . . . . . . 9
3.1 Query and Reporting . . . . . . . . . . . . . . . . . . . . . . . . . . . . . . . . 10
3.2 Multidimensional Analysis . . . . . . . . . . . . . . . . . . . . . . . . . . . . 11
3.3 Data Mining . . . . . . . . . . . . . . . . . . . . . . . . . . . . . . . . . . . . . 12
3.4 Imprtance to Modeling . . . . . . . . . . . . . . . . . . . . . . . . . . . . . . 13
Chapter 4. Data Warehousing Architecture and Implementation Choices . . . 15
4.1 Architecture Choices . . . . . . . . . . . . . . . . . . . . . . . . . . . . . . . . 15
4.1.1 Global Warehouse Architecture . . . . . . . . . . . . . . . . . . . . . . . 15
4.1.2 Independent Data Mart Architecture . . . . . . . . . . . . . . . . . . . . 17
4.1.3 Interconnected Data Mart Architecture . . . . . . . . . . . . . . . . . . 18
4.2 Implementation Choices . . . . . . . . . . . . . . . . . . . . . . . . . . . . . . 18
4.2.1 Top Down Implementation . . . . . . . . . . . . . . . . . . . . . . . . . . 19
4.2.2 Bottom Up Implementation . . . . . . . . . . . . . . . . . . . . . . . . . 20
4.2.3 A Combined Approach . . . . . . . . . . . . . . . . . . . . . . . . . . . . 21
Chapter 5. Architecting the Data . . . . . . . . . . . . . . . . . . . . . . . . . . . 23
5.1 Structuring the Data . . . . . . . . . . . . . . . . . . . . . . . . . . . . . . . . 23
5.1.1 Real-Time Data . . . . . . . . . . . . . . . . . . . . . . . . . . . . . . . . 24
5.1.2 Derived Data . . . . . . . . . . . . . . . . . . . . . . . . . . . . . . . . . . 24
5.1.3 Reconciled Data . . . . . . . . . . . . . . . . . . . . . . . . . . . . . . . . 24
5.2 Enterprise Data Model . . . . . . . . . . . . . . . . . . . . . . . . . . . . . . . 25
5.2.1 Phased Enterprise Data Modeling . . . . . . . . . . . . . . . . . . . . . 25
5.2.2 A Simple Enterprise Data Model . . . . . . . . . . . . . . . . . . . . . . 26
5.2.3 The Benefits of EDM . . . . . . . . . . . . . . . . . . . . . . . . . . . . . 27
5.3 Data Granularity Model . . . . . . . . . . . . . . . . . . . . . . . . . . . . . . 28
5.3.1 Granularity of Data in the Data Warehouse . . . . . . . . . . . . . . . . 28
5.3.2 Multigranularity Modeling in the Corporate Environment . . . . . . . 30
5.4 Logical Data Partitioning Model . . . . . . . . . . . . . . . . . . . . . . . . . 30
5.4.1 Partitioning the Data . . . . . . . . . . . . . . . . . . . . . . . . . . . . . 31
5.4.1.1 The Goals of Partitioning . . . . . . . . . . . . . . . . . . . . . . . . 31
5.4.1.2 The Criteria of Partitioning . . . . . . . . . . . . . . . . . . . . . . . 31
5.4.2 Subject Area . . . . . . . . . . . . . . . . . . . . . . . . . . . . . . . . . . 32
Chapter 6. Data Modeling for a Data Warehouse . . . . . . . . . . . . . . . . . 35
6.1 Why Data Modeling Is Imprtant . . . . . . . . . . . . . . . . . . . . . . . . . 35
Visualization of the business world . . . . . . . . . . . . . . . . . . . . . 35
The essence of the data warehouse architecture . . . . . . . . . . . . 36
Different approaches of data modeling . . . . . . . . . . . . . . . . . . . 36
6.2 Data Modeling Techniques . . . . . . . . . . . . . . . . . . . . . . . . . . . . 36
6.3 ER Modeling . . . . . . . . . . . . . . . . . . . . . . . . . . . . . . . . . . . . . 37
6.3.1 Basic Concepts . . . . . . . . . . . . . . . . . . . . . . . . . . . . . . . . 37
6.3.1.1 Entity . . . . . . . . . . . . . . . . . . . . . . . . . . . . . . . . . . . . 37
6.3.1.2 Relationship . . . . . . . . . . . . . . . . . . . . . . . . . . . . . . . . 38
6.3.1.3 Attributes . . . . . . . . . . . . . . . . . . . . . . . . . . . . . . . . . 38
6.3.1.4 Other Concepts . . . . . . . . . . . . . . . . . . . . . . . . . . . . . . 39
6.3.2 Advanced Topics in ER Modeling . . . . . . . . . . . . . . . . . . . . . . 39
6.3.2.1 Supertype and Subtype . . . . . . . . . . . . . . . . . . . . . . . . . 39
6.3.2.2 Constraints . . . . . . . . . . . . . . . . . . . . . . . . . . . . . . . . 40
6.3.2.3 Derived Attributes and Derivation Functions . . . . . . . . . . . . 41
6.4 Dimensional Modeling . . . . . . . . . . . . . . . . . . . . . . . . . . . . . . . 42
6.4.1 Basic Concepts . . . . . . . . . . . . . . . . . . . . . . . . . . . . . . . . 42
6.4.1.1 Fact . . . . . . . . . . . . . . . . . . . . . . . . . . . . . . . . . . . . . 42
6.4.1.2 Dimension . . . . . . . . . . . . . . . . . . . . . . . . . . . . . . . . . 42
Dimension Members . . . . . . . . . . . . . . . . . . . . . . . . . . . . . . 43
Dimension Hierarchies . . . . . . . . . . . . . . . . . . . . . . . . . . . . 43
6.4.1.3 Measure . . . . . . . . . . . . . . . . . . . . . . . . . . . . . . . . . . 43
6.4.2 Visualization of a Dimensional Model . . . . . . . . . . . . . . . . . . . 43
6.4.3 Basic Operations for OLAP . . . . . . . . . . . . . . . . . . . . . . . . . 44
6.4.3.1 Drill Down and Roll Up . . . . . . . . . . . . . . . . . . . . . . . . . 44
6.4.3.2 Slice and Dice . . . . . . . . . . . . . . . . . . . . . . . . . . . . . . 45
6.4.4 Star and Snowflake Models . . . . . . . . . . . . . . . . . . . . . . . . . 45
6.4.4.1 Star Model . . . . . . . . . . . . . . . . . . . . . . . . . . . . . . . . 46
6.4.4.2 Snowflake Model . . . . . . . . . . . . . . . . . . . . . . . . . . . . . 46
6.4.5 Data Consolidation . . . . . . . . . . . . . . . . . . . . . . . . . . . . . . 47
6.5 ER Modeling and Dimensional Modeling . . . . . . . . . . . . . . . . . . . . 47
Chapter 7. The Process of Data Warehousing . . . . . . . . . . . . . . . . . . . 49
7.1 Manage the Project . . . . . . . . . . . . . . . . . . . . . . . . . . . . . . . . 50
7.2 Define the Project . . . . . . . . . . . . . . . . . . . . . . . . . . . . . . . . . 51
7.3 Requirements Gathering . . . . . . . . . . . . . . . . . . . . . . . . . . . . . 51
7.3.1 Source-Driven Requirements Gathering . . . . . . . . . . . . . . . . . . 52
7.3.2 User-Driven Requirements Gathering . . . . . . . . . . . . . . . . . . . 53
7.3.3 The CelDial Case Study . . . . . . . . . . . . . . . . . . . . . . . . . . . 53
7.4 Modeling the Data Warehouse . . . . . . . . . . . . . . . . . . . . . . . . . . 53
7.4.1 Creating an ER Model . . . . . . . . . . . . . . . . . . . . . . . . . . . . 54
7.4.2 Creating a Dimensional Model . . . . . . . . . . . . . . . . . . . . . . . 55
7.4.2.1 Dimensions and Measures . . . . . . . . . . . . . . . . . . . . . . . 55
7.4.2.2 Adding a Time Dimension . . . . . . . . . . . . . . . . . . . . . . . 57
7.4.2.3 Creating Facts . . . . . . . . . . . . . . . . . . . . . . . . . . . . . . 58
7.4.2.4 Granularity, Additivity, and Merging Facts . . . . . . . . . . . . . . 58
Granularity and Additivity . . . . . . . . . . . . . . . . . . . . . . . . . . . 60
Fact Consolidation . . . . . . . . . . . . . . . . . . . . . . . . . . . . . . . 60
7.4.2.5 Integration with Existing Models . . . . . . . . . . . . . . . . . . . . 64
7.4.2.6 Sizing Your Model . . . . . . . . . . . . . . . . . . . . . . . . . . . . 65
7.4.3 Don′t Forget the Metadata . . . . . . . . . . . . . . . . . . . . . . . . . . 66
7.4.4 Validating the Model . . . . . . . . . . . . . . . . . . . . . . . . . . . . . 68
7.5 Design the Warehouse . . . . . . . . . . . . . . . . . . . . . . . . . . . . . . . 69
7.5.1 Data Warehouse Design versus Operational Design . . . . . . . . . . 69
7.5.2 Identifying the Sources . . . . . . . . . . . . . . . . . . . . . . . . . . . . 71
7.5.3 Cleaning the Data . . . . . . . . . . . . . . . . . . . . . . . . . . . . . . . 72
7.5.4 Transforming the Data . . . . . . . . . . . . . . . . . . . . . . . . . . . . 72
7.5.4.1 Capturing the Source Data . . . . . . . . . . . . . . . . . . . . . . . 73
7.5.4.2 Generating Keys . . . . . . . . . . . . . . . . . . . . . . . . . . . . . 73
7.5.4.3 Getting from Source to Target . . . . . . . . . . . . . . . . . . . . . 74
7.5.5 Designing Subsidiary Targets . . . . . . . . . . . . . . . . . . . . . . . . 76
7.5.6 Validating the Design . . . . . . . . . . . . . . . . . . . . . . . . . . . . . 77
7.5.7 What About Data Mining? . . . . . . . . . . . . . . . . . . . . . . . . . . 77
7.5.7.1 Data Scoping . . . . . . . . . . . . . . . . . . . . . . . . . . . . . . . 78
7.5.7.2 Data Selection . . . . . . . . . . . . . . . . . . . . . . . . . . . . . . 78
7.5.7.3 Data Cleaning . . . . . . . . . . . . . . . . . . . . . . . . . . . . . . 78
7.5.7.4 Data Transformation . . . . . . . . . . . . . . . . . . . . . . . . . . . 79
7.5.7.5 Data Summarization . . . . . . . . . . . . . . . . . . . . . . . . . . . 79
7.6 The Dynamic Warehouse Model . . . . . . . . . . . . . . . . . . . . . . . . . 79
Chapter 8. Data Warehouse Modeling Techniques . . . . . . . . . . . . . . . . 81
8.1 Data Warehouse Modeling and OLTP Database Modeling . . . . . . . . . 81
8.1.1 Origin of the Modeling Differences . . . . . . . . . . . . . . . . . . . . . 82
8.1.2 Base Properties of a Data Warehouse . . . . . . . . . . . . . . . . . . . 82
8.1.3 The Data Warehouse Computing Context . . . . . . . . . . . . . . . . . 84
8.1.4 Setting Up a Data Warehouse Modeling Approach . . . . . . . . . . . 85
8.2 Principal Data Warehouse Modeling Techniques . . . . . . . . . . . . . . . 86
8.3 Data Warehouse Modeling for Data Marts . . . . . . . . . . . . . . . . . . . 86
8.4 Dimensional Modeling . . . . . . . . . . . . . . . . . . . . . . . . . . . . . . . 88
8.4.1 Requirements Gathering . . . . . . . . . . . . . . . . . . . . . . . . . . . 92
8.4.1.1 Process Oriented Requirements . . . . . . . . . . . . . . . . . . . 93
8.4.1.2 Information-Oriented Requirements . . . . . . . . . . . . . . . . . 95
8.4.2 Requirements Analysis . . . . . . . . . . . . . . . . . . . . . . . . . . . . 96
8.4.2.1 Determining Candidate Measures, Dimensions, and Facts . . . . 98
Candidate Measures . . . . . . . . . . . . . . . . . . . . . . . . . . . . . . 98
Candidate Dimensions . . . . . . . . . . . . . . . . . . . . . . . . . . . . 99
Candidate Facts . . . . . . . . . . . . . . . . . . . . . . . . . . . . . . . 100
8.4.2.2 Creating the Initial Dimensional Model . . . . . . . . . . . . . . 105
Establishing the Business Directory . . . . . . . . . . . . . . . . . . . 105
Determining Facts and Dimension Keys . . . . . . . . . . . . . . . . . 106
Determining Representative Dimensions and Detailed Versus
Consolidated Facts . . . . . . . . . . . . . . . . . . . . . . . . . . . . . 109
Dimensions and Their Roles in a Dimensional Model . . . . . . . . . 111
Getting the Measures Right . . . . . . . . . . . . . . . . . . . . . . . . 112
Fact Attributes Other Than Dimension Keys and Measures . . . . . 114
8.4.3 Requirements Validation . . . . . . . . . . . . . . . . . . . . . . . . . . 115
8.4.4 Requirements Modeling - CelDial Case Study Example . . . . . . . 117
8.4.4.1 Modeling of Nontemporal Dimensions . . . . . . . . . . . . . . . 120
The Product Dimension . . . . . . . . . . . . . . . . . . . . . . . . . . . 121
Analyzing the Extended Product Dimension . . . . . . . . . . . . . 123
Looking for Fundamental Aggregation Paths . . . . . . . . . . . . . 124
The Manufacturing Dimension . . . . . . . . . . . . . . . . . . . . . . . 125
The Customer Dimension . . . . . . . . . . . . . . . . . . . . . . . . . . 126
The Sales Organization Dimension . . . . . . . . . . . . . . . . . . . . 126
The Time Dimension . . . . . . . . . . . . . . . . . . . . . . . . . . . . . 127
8.4.4.2 Developing the Basis of a Time Dimension Model . . . . . . . . 127
About Aggregation Paths above Week . . . . . . . . . . . . . . . . . . 128
Business Time Periods and Business-Related Time Attributes . . 130
Making the Time Dimension Model More Generic . . . . . . . . . . . 131
Flattening the Time Dimension Model into a Dimension Table . . . 132
The Time Dimension As a Means for Consistency . . . . . . . . . . . 132
Lower Levels of Time Granularity . . . . . . . . . . . . . . . . . . . . . 133
8.4.4.3 Modeling Slow-Varying Dimensions . . . . . . . . . . . . . . . . 133
About Keys in Dimensions of a Data Warehouse . . . . . . . . . . . . 133
Dealing with Attribute Changes in Slow-Varying Dimensions . . . . 135
Modeling Time-Variancy of the Dimension Hierarchy . . . . . . . . . 137
8.4.4.4 Temporal Data Modeling . . . . . . . . . . . . . . . . . . . . . . . 139
Preliminary Considerations . . . . . . . . . . . . . . . . . . . . . . . . . 141
Time Stamp Interpretations . . . . . . . . . . . . . . . . . . . . . . . 143
Instant and Interval Time Stamps . . . . . . . . . . . . . . . . . . . 144
Base Temporal Modeling Techniques . . . . . . . . . . . . . . . . . . 145
Adding Time Stamps to Entities . . . . . . . . . . . . . . . . . . . . 145
Restructuring the Entities . . . . . . . . . . . . . . . . . . . . . . . . 146
Adding Entities for Transactions and Events . . . . . . . . . . . . . 148
Grouping Time-Variant Classes of Attributes . . . . . . . . . . . . 149
Advanced Temporal Modeling Techniques . . . . . . . . . . . . . . . 149
Adding Temporal Constraints to a Model . . . . . . . . . . . . . . . 149
Modeling Lifespan Histories of Database Objects . . . . . . . . . . 150
Modeling Time-Variancy at the Schema Level . . . . . . . . . . . . 150
Some Conclusions . . . . . . . . . . . . . . . . . . . . . . . . . . . . . . 150
8.4.4.5 Selecting a Data Warehouse Modeling Approach . . . . . . . . 151
Considerations for ER Modeling . . . . . . . . . . . . . . . . . . . . . . 152
Considerations for Dimensional Modeling . . . . . . . . . . . . . . . . 152
Two-Tiered Data Modeling . . . . . . . . . . . . . . . . . . . . . . . . . 152
Dimensional Modeling Supporting Drill Across . . . . . . . . . . . . . 153
Modeling Corporate Historical Databases . . . . . . . . . . . . . . . . 153
Chapter 9. Selecting a Modeling Tool . . . . . . . . . . . . . . . . . . . . . . . 155
9.1 Diagram Notation . . . . . . . . . . . . . . . . . . . . . . . . . . . . . . . . . 155
9.1.1 ER Modeling . . . . . . . . . . . . . . . . . . . . . . . . . . . . . . . . . 155
9.1.2 Dimensional Modeling . . . . . . . . . . . . . . . . . . . . . . . . . . . 156
9.2 Reverse Engineering . . . . . . . . . . . . . . . . . . . . . . . . . . . . . . . 156
9.3 Forward Engineering . . . . . . . . . . . . . . . . . . . . . . . . . . . . . . . 156
9.4 Source to Target Mapping . . . . . . . . . . . . . . . . . . . . . . . . . . . 157
9.5 Data Dictionary (Repository) . . . . . . . . . . . . . . . . . . . . . . . . . . 157
9.6 Reporting . . . . . . . . . . . . . . . . . . . . . . . . . . . . . . . . . . . . . 158
9.7 Tools . . . . . . . . . . . . . . . . . . . . . . . . . . . . . . . . . . . . . . . . 158
Chapter 10. Populating the Data Warehouse . . . . . . . . . . . . . . . . . . . 159
10.1 Capture . . . . . . . . . . . . . . . . . . . . . . . . . . . . . . . . . . . . . . 159
10.2 Transform . . . . . . . . . . . . . . . . . . . . . . . . . . . . . . . . . . . . . 161
10.3 Apply . . . . . . . . . . . . . . . . . . . . . . . . . . . . . . . . . . . . . . . 161
10.4 Imprtance to Modeling . . . . . . . . . . . . . . . . . . . . . . . . . . . . 162
Appendix A. The CelDial Case Study . . . . . . . . . . . . . . . . . . . . . . . 163
A.1 CelDial - The Company . . . . . . . . . . . . . . . . . . . . . . . . . . . . . 163
A.2 Project Definition . . . . . . . . . . . . . . . . . . . . . . . . . . . . . . . . . 163
A.3 Defining the Business Need . . . . . . . . . . . . . . . . . . . . . . . . . . 164
A.3.1 Life Cycle of a Product . . . . . . . . . . . . . . . . . . . . . . . . . . . 164
A.3.2 Anatomy of a Sale . . . . . . . . . . . . . . . . . . . . . . . . . . . . . 165
A.3.3 Structure of the Organization . . . . . . . . . . . . . . . . . . . . . . . 165
A.3.4 Defining Cost and Revenue . . . . . . . . . . . . . . . . . . . . . . . . 165
A.3.5 What Do the Users Want? . . . . . . . . . . . . . . . . . . . . . . . . . 166
A.4 Getting the Data . . . . . . . . . . . . . . . . . . . . . . . . . . . . . . . . . 167
A.5 CelDial Dimensional Models - Proposed Solution . . . . . . . . . . . . . 167
A.6 CelDial Metadata - Proposed Solution . . . . . . . . . . . . . . . . . . . . 170
Appendix B. Special Notices . . . . . . . . . . . . . . . . . . . . . . . . . . . . 183
Appendix C. Related Publications . . . . . . . . . . . . . . . . . . . . . . . . . 185
C.1 International Technical Support Organization Publications . . . . . . . . 185
C.2 Redbooks on CD-ROMs . . . . . . . . . . . . . . . . . . . . . . . . . . . . . 185
C.3 Other Publications . . . . . . . . . . . . . . . . . . . . . . . . . . . . . . . . 185
C.3.1 Books . . . . . . . . . . . . . . . . . . . . . . . . . . . . . . . . . . . . . 185
C.3.2 Journal Articles, Technical Reports, and Miscellaneous Sources . 186
How to Get ITSO Redbooks . . . . . . . . . . . . . . . . . . . . . . . . . . . . . 189
How IBM Employees Can Get ITSO Redbooks . . . . . . . . . . . . . . . . . . 189
How Customers Can Get ITSO Redbooks . . . . . . . . . . . . . . . . . . . . . 190
IBM Redbook Order Form . . . . . . . . . . . . . . . . . . . . . . . . . . . . . . 191
Glossary . . . . . . . . . . . . . . . . . . . . . . . . . . . . . . . . . . . . . . . . . 193
Index . . . . . . . . . . . . . . . . . . . . . . . . . . . . . . . . . . . . . . . . . . . 195
ITSO Redbook Evaluation . . . . . . . . . . . . . . . . . . . . . . . . . . . . . . . 197
Other Data Warehouse Books
Download
No comments:
Post a Comment